Predicting the climate with machine learning
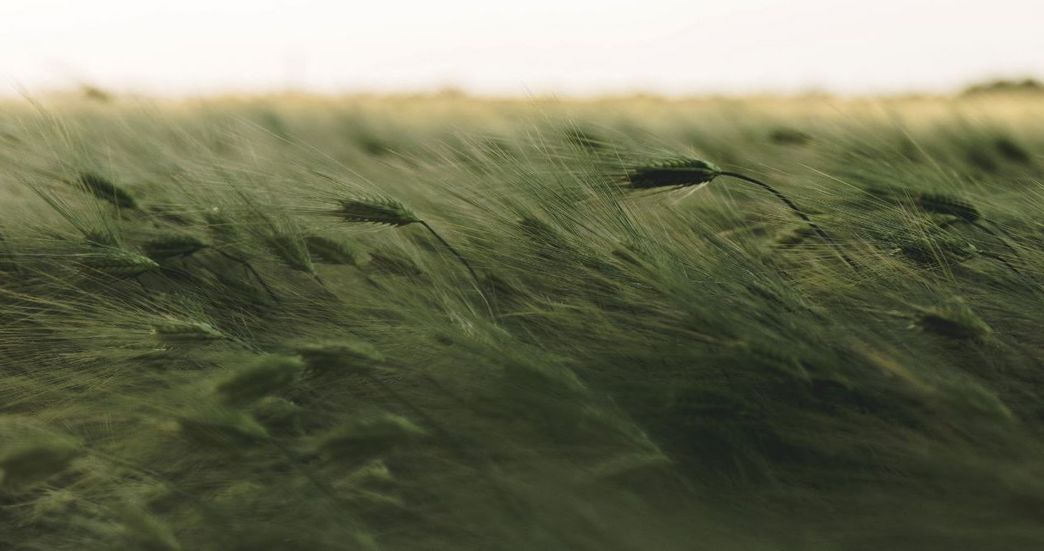
Could the surface temperature in the tropical Atlantic be one of the causes of droughts in the Mediterranean region? With the help of machine learning, Eduardo Zorita at the Helmholtz Centre for Materials and Coastal Research in Geesthacht (HZG) is trying to find out which factors have an impact on the climate.
Spain and Italy experienced a devastating drought summer in 2017. Almonds and olives shrank, and grain yields dropped to their lowest levels in twenty years. How can climate research prepare people for such states of emergency?
By predicting as accurately as possible what is to come. At the Department of Climate Impact Research at HZG we are working on models and simulations of climate dynamics. They show, for example, how storms develop and make seasonal predictions of water levels and the rise in sea levels on the coasts. I myself work on drought forecasts in the Mediterranean region.
Can you explain this in more detail?
Whether we will experience a drought in the summer is determined by the previous winter. Eighty percent of precipitation falls during this period. If little rain falls between October and March, a drought can be expected the following summer because the soil is dry down to its lower depths, and water levels in reservoirs have not survived the warmer months. Our idea is that if we are able to predict in the previous summer how much precipitation there will be in the winter, farmers could get prepared in time, for example, by buying drought-resistant crops. When droughts are to be expected, other industries could adjust their water use accordingly, for example, by using less hydropower. And droughts are going to increase rapidly in many regions in the coming years, according to the Intergovernmental Panel on Climate Change (IPCC). We need climate models that make forecasts that are as reliable as possible. This is a very exciting field in which there is still much to be done.
How did you get into climate research?
I grew up with the natural sciences. My mother was a biologist and my father a veterinarian. We often discussed scientific topics at the kitchen table. I remember my mother telling me about working with cell cultures, why you do it, how you feed the cultures, what you can find in them using a microscope. My father used to explain me the correct way of feeding cows, what they need to eat so that they have higher yields, give good milk and stay healthy for a long time. As a teenager I had a math teacher who explored astronomy with a small group of us kids. Sometimes we would meet at night and take pictures of celestial bodies with the professional telescope of our school ...
... sounds a little like Dead Poets Society, the cult movie with Robin Williams ...
... it had a little something of that, yes. I was passionate about physics back then. I studied in Zaragoza and ended up getting my PhD in Solid State Physics, which had a particularly good reputation there. But in the long run, it wasn’t for me. Too much laboratory work, taking measurements, and it was too tricky for my hands and too technical for me.
Regional phenomena can influence the global climate
A scholarship from the German Academic Exchange Service (DAAD) brought you to the Max Planck Institute for Meteorology in Hamburg in the mid-1980s.
The climatologist Josef Oberhuber had given a tremendously impressive lecture there on the El Niño ocean current and its influence on the sudden warming of the tropical Pacific. That was when I understood just how much regional phenomena can influence the global climate. It was a key moment for me. I knew that was where I wanted to do my work. I hung up the lab coat and swapped it for an office chair and a PC, although I had no idea about data analysis and climate models. A government research grant from Spain made it all possible. I went to the library day and night to get my head around the material. My first topic was the causes of droughts in Spain and the climate models that can be used to predict periods of droughts. Incredibly exciting.
What makes climate research so interesting?
It’s an extremely complex, multifaceted field. Physics, chemistry, meteorology, biology, data sciences, it’s all part of it. An enormous number of parameters influence each other. Later, it also became the social responsibility and questions about the social consequences of climate change that motivated me to get involved in these research questions. You can make a big impact.
For a while you were also concentrating on the paleoclimate, the climate of the past. What is that about?
We are studying climate changes over the past 2,000 years. We ask questions like: What variations exist, how are they related to human behavior, to solar activity, to volcanic eruptions? Which factor played what part? In paleoclimate research we work closely with historians and thus constantly come across interesting correlations.
Such as?
500 years ago, for example, there was a small ice age on Earth that was also reflected in art. Dutch painters often painted frozen lakes and snowy landscapes. The spread of the plague was probably also favored by the climatic changes. Similarly, the expansion of the Roman Empire is hardly conceivable without a climatically extremely mild phase. For instance, this was the only thing that made it possible to feed the troops in North Africa without any problem. Grain grew there at the time, and Italy was even importing it.
Today you are back to researching on your original topic of drought forecasts for the Mediterranean region at the Institute for Materials and Coastal Research in Geesthacht.
Yes, we’re working on climate models that should give us the most accurate predictions possible. So far, however, we are still at the very beginning.
The capacity of today’s computers is not nearly sufficient for the amount of artificially generated data.
What makes it so difficult?
We work with simulations that have to generate and process incredible amounts of data themselves. To do this, we feed key data into the system on the temperature of sea surfaces, sea ice, snow cover, soil and air humidity, weather, geographical data on the landscape and so on. This is observational data. Based on this, the system generates new data and calculates a simulation. But the capacity of today’s computers does not even begin to cover the amount of artificially generated data we would need to be able to reproduce reality adequately.
So the resolution of the climate models is extremely coarse?
Exactly. The altitude of the Alps, for example, is represented by just a few figures. If you use a coordinate grid in a simulation, which is supposed to represent about 200 km, and zoom in closer, the Alps are therefore just a lump without details. Clouds do not appear at all; they are much too small. Of course, we could simulate great images of the Alps with x weather, soil and climate data. But then they only show a miniature section, with which no climate predictions can be made. In addition, the simulation models are not yet technically perfect and lots of data are still incomplete. We would need data as complete as possible on winds, sea temperatures at different depths, cloud cover, humidity and so on, which are continuously collected over time. But so far there are only selective measurements.
What solutions do you foresee in the future?
We hope that AI-based models will move us considerably forward. The decisive advantage of Big Data applications based on machine learning is that you can ask the system a very specific question, which requires less data to process than hypercomplex simulations. Moreover, machine learning algorithms work quite differently than simulations. They search empirically for patterns in data, for correlations, and ideally, they even find causal relationships. Could one of the causes of the drought in the Mediterranean region perhaps be the sea surface temperatures in the tropical Atlantic? Or the ice cover in the Arctic Ocean? Machine learning could replace or significantly improve our statistical methods so that droughts no longer turn into disasters.
Interview: Anja Dilk
"Machine learning could replace or significantly improve our statistical methods. So that droughts no longer become disasters."
Eduardo Zorita